Crafting a Data Science Success Story: 11 Key Topics to Engage Stakeholders Effectively
Discover the essential elements to transform a data science project into a compelling narrative for stakeholders. From business use cases to revenue reconciliation, learn how to align technical insights with business goals effectively.
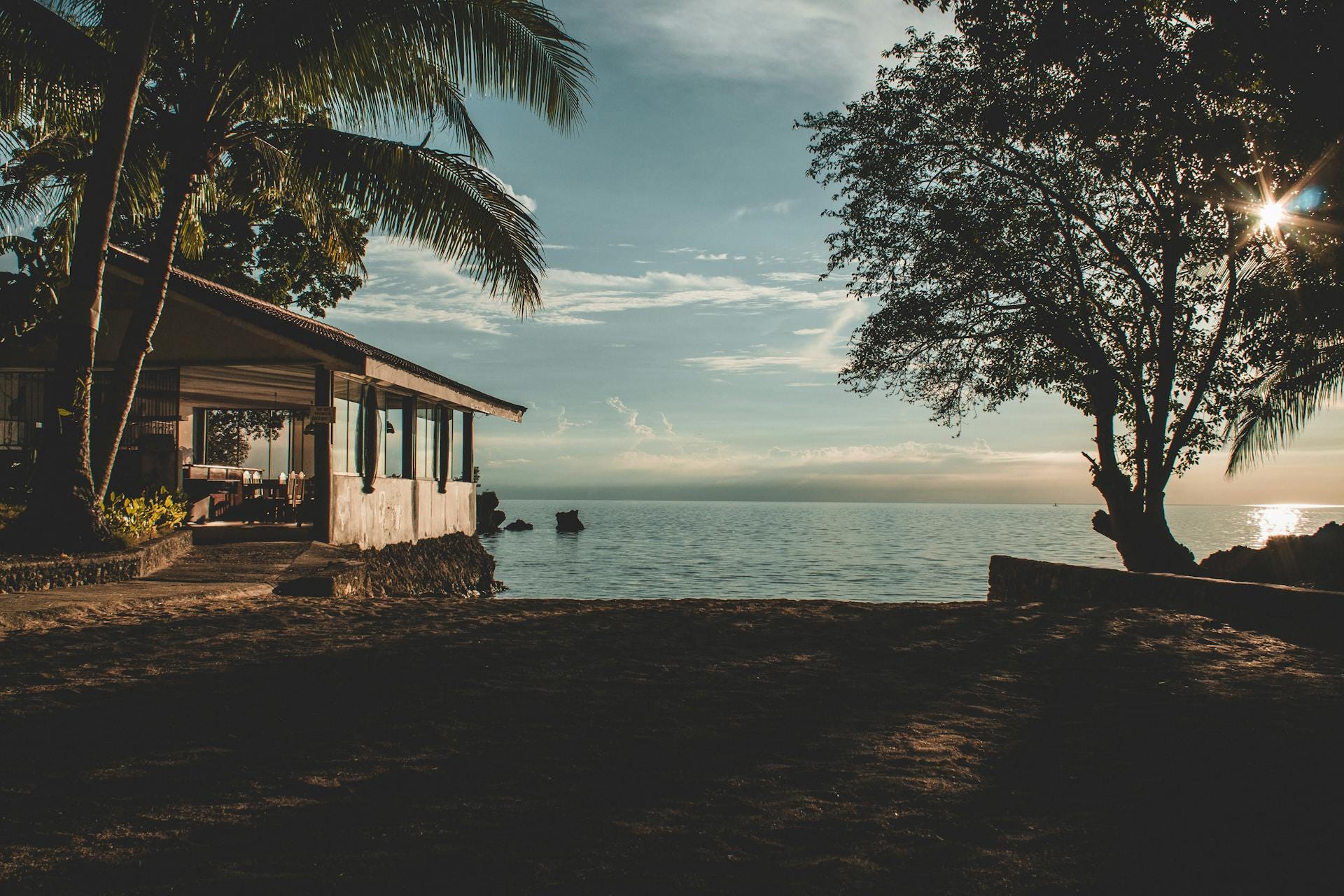
Hi everyone! While data science itself is essential, effectively managing data science projects is equally crucial in shaping a successful data science journey.
Let’s dive into the key topics that need to be prioritized to turn the data science narrative into a true success story for stakeholders.
- Title
- Business Use-case
- Challenges & Solution
- Workflow
- Project Timeline
- Exploratory Data Analysis
- Attributes used
- Model used
- Model Evaluation
- Business Model Evaluation(from previous historical accuracy)
- Revenue Reconciliation
To ensure clarity, impact, and alignment with business goals, it’s essential to focus on specific areas that shape the project’s narrative. Let’s understand each of them a bit in brief.
1. Business Use Case
Begin by defining the business problem or opportunity the project aims to address. This section should outline how the data science initiative aligns with the organization’s objectives, such as increasing efficiency, improving customer experience, or driving revenue.
2. Challenges and Solutions
Identify the challenges encountered during the project, such as data availability, quality issues, or integration with existing systems. Pair these with the solutions implemented to overcome them, demonstrating the team’s problem-solving approach and adaptability.
3. Workflow
Present the workflow of the project, illustrating the step-by-step process followed, from data collection to model deployment. Visual aids like flowcharts can simplify this for stakeholders, providing a clear view of the project’s structure.
You can use this work great! https://app.diagrams.net/
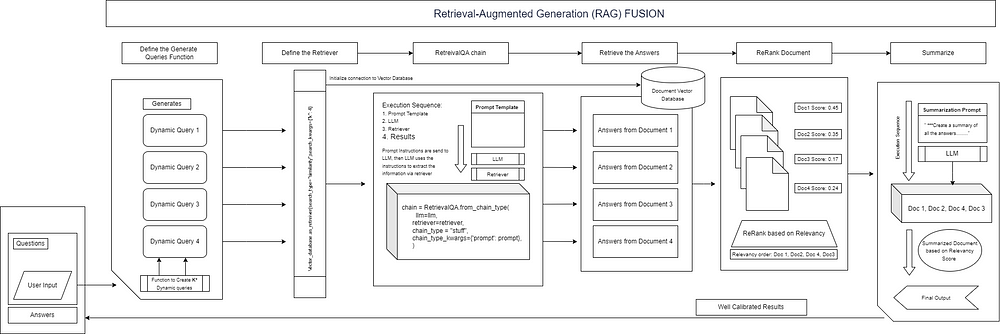
Revolutionize the way we approach natural language processing and information retrieval. We delve into the intricacies…bobrupakroy.medium.com
4. Project Timeline
Lay out the timeline of the project, highlighting key milestones and deliverables. This provides stakeholders with an understanding of the project’s scope and ensures alignment on deadlines and expectations.
5. Exploratory Data Analysis (EDA)
Showcase the EDA phase by summarizing key insights derived from the data. This might include patterns, trends, and anomalies that informed the next steps of the project. Visualizations like charts and heatmaps are highly effective in this section.
6. Attributes Used
Highlight the specific attributes (features) used for the analysis or model training. Explain why these attributes were chosen and their significance in solving the business problem.
7. Model Used
Detail the model or algorithms selected for the project, such as regression, classification, or deep learning techniques. Include a brief explanation of why this model was the best fit for the problem at hand.
8. Model Evaluation
Provide an overview of the model evaluation metrics used, such as accuracy, precision, recall, or F1-score. These metrics should demonstrate the model’s reliability and effectiveness in addressing the business use case.
9. Business Model Evaluation
Compare the model’s performance with historical data to evaluate its alignment with past accuracy and trends. This comparison helps stakeholders understand how the model contributes to business decisions based on proven success.
Example: Previously, the accuracy, whether with or without a model, stood at 25% over a span of 4–5 months. However, the current machine learning model has achieved a significant improvement, delivering 45% accuracy over the same timeframe. This reflects a substantial 20% increase/lift in accuracy, with the potential for further growth over time.
10. Revenue Reconciliation
Illustrate the financial impact of the project by reconciling the predicted outcomes with revenue or cost savings. Highlight how the insights or models drive measurable business value, reinforcing the project’s importance.
That’s it by prioritizing these topics in your narrative, you create a clear, impactful, and results-driven presentation that resonates with stakeholders. This approach ensures alignment with business goals, builds confidence in the project’s outcomes, and lays the foundation for future data science initiatives.
Next, we will try to understand and explore ways to manage a data science project as well the team.
Thanks for your time, if you enjoyed this short article there are tons of topics in advanced analytics, data science, and machine learning available in my medium repo. https://medium.com/@bobrupakroy
Some of my alternative internet presences are Facebook, Instagram, Udemy, Blogger, Issuu, Slideshare, Scribd, and more.
Also available on Quora @ https://www.quora.com/profile/Rupak-Bob-Roy
Let me know if you need anything. Talk Soon.
Check out the links i hope it helps.
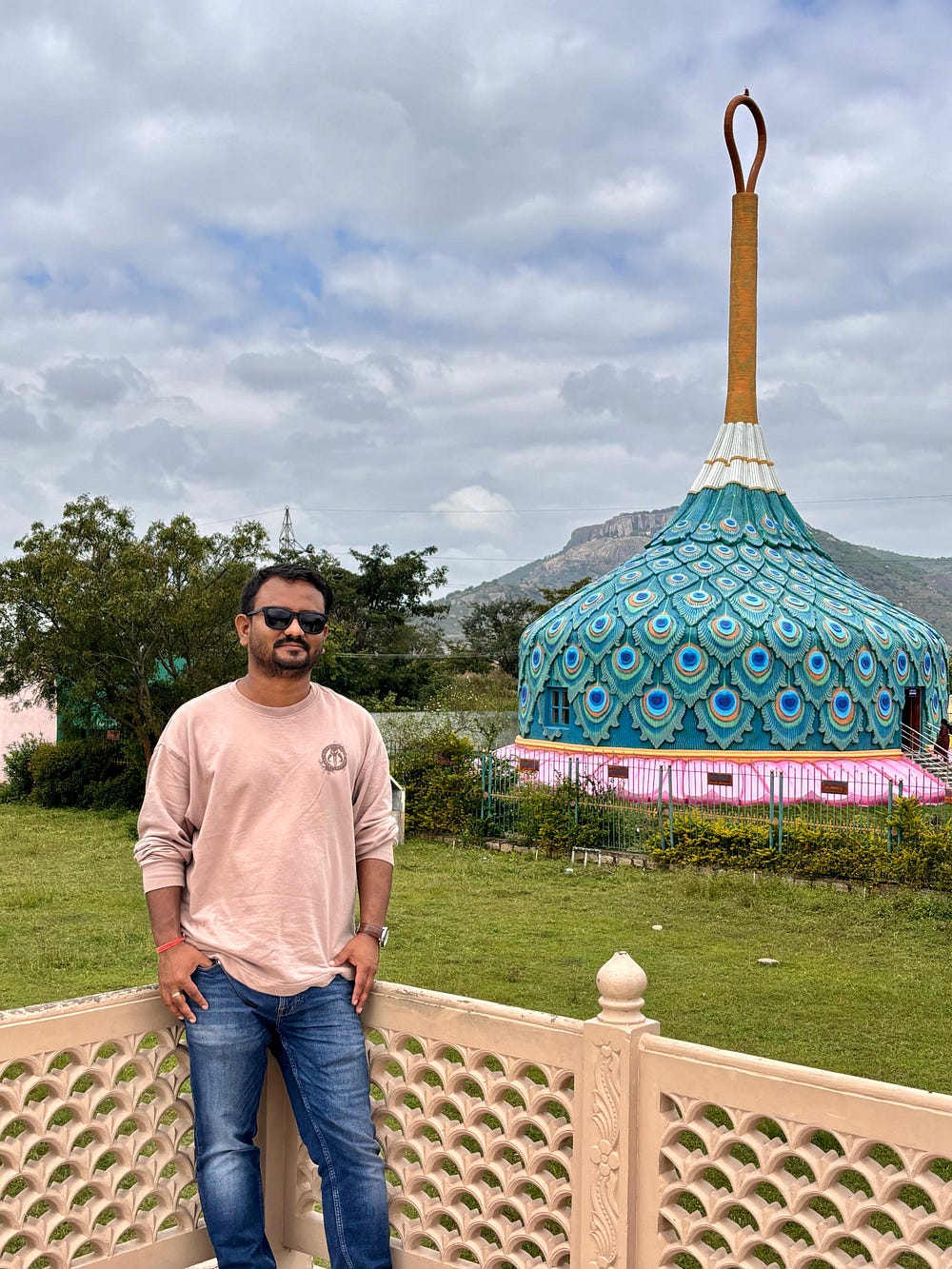
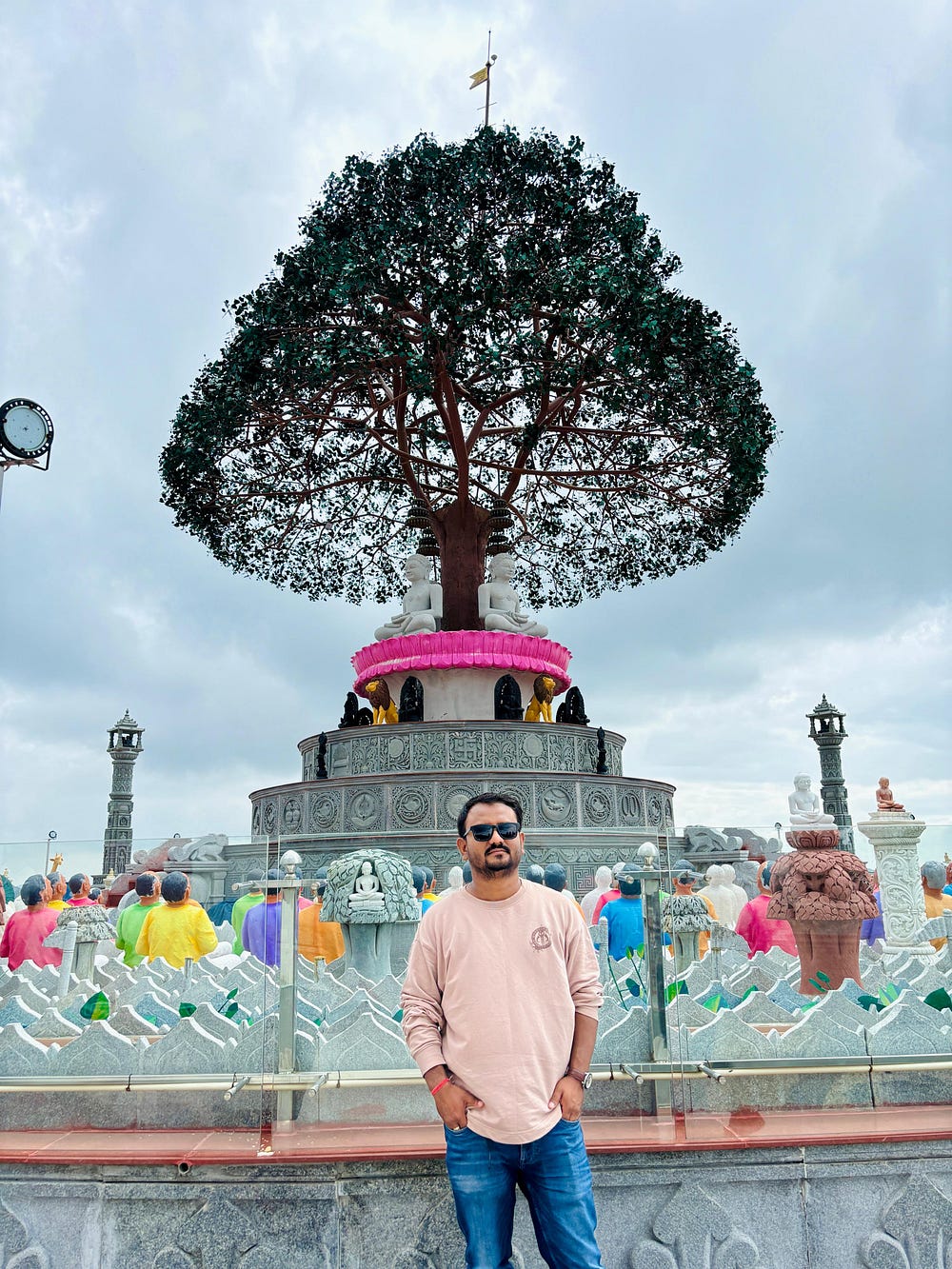
Nice place to visit Tumakuru, Karnataka
Comments
Post a Comment