Machine Capable of Creating Thinking AiArt | Create your own Mid-journey via VQGAN+CLIP
Machine Capable of Creating Thinking AiArt
Create your own Mid-journey via VQGAN+CLIP
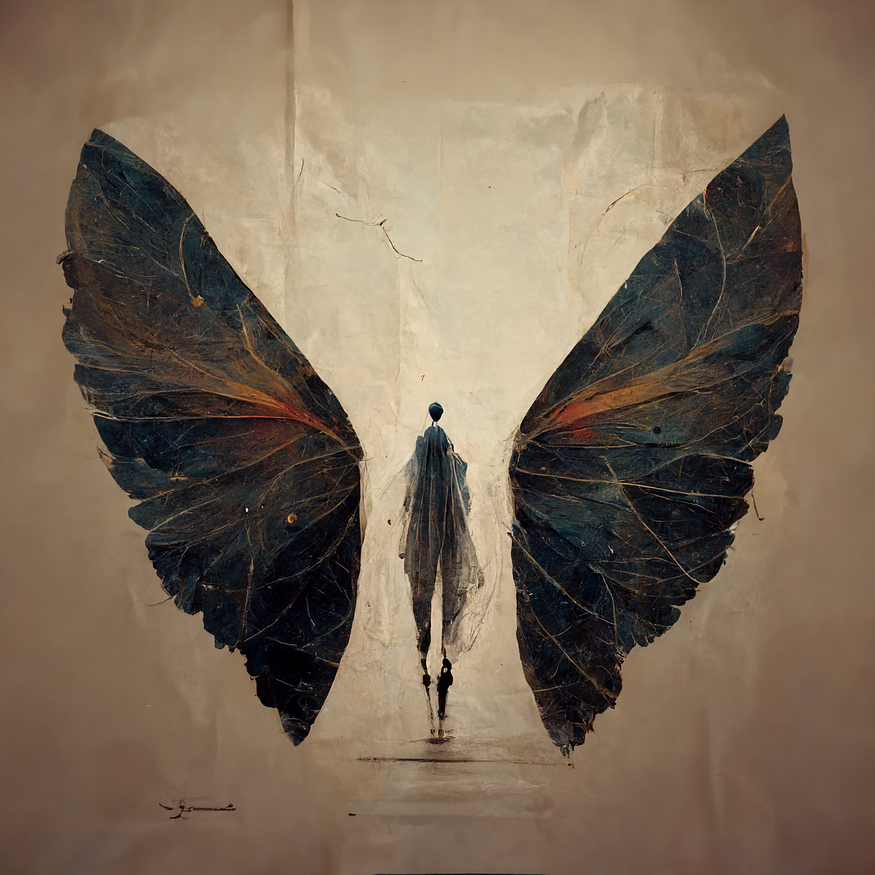
Hi there, it's been a while since i wrote something new and trending, I thought the topic of stable diffusion will be a great topic.
Let’s get started creating our own mid-journey similar application, I will keep it short and simple.
We will be using VQGAN and CLIP combined.
So let’s start VQGAN in brief:
VQGAN stands for Vector Quantized Generative Adversarial Network, while CLIP stands for Contrastive Image-Language Pretraining. Whenever we say VQGAN-CLIP1, we refer to the interaction between these two networks. They’re separate models that work in tandem. The way they work is that VQGAN generates the images, while CLIP judges how well an image matches our text prompt. This interaction guides our generator to produce more accurate images.
VQGAN employs a two-stage structure by learning an intermediary representation before feeding it to a transformer. However, instead of downsampling the image, VQGAN uses a codebook to represent visual parts. The authors did not model the image from a pixel-level directly, but instead from the codewords of the learned codebook.
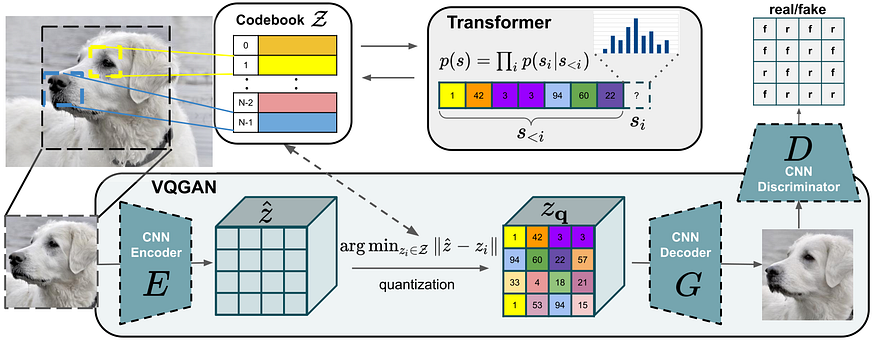
VQGAN was able to solve Transformer’s scaling problem by using an intermediate representation known as a codebook. This codebook serves as the bridge for the two-stage approach found in most image transformer techniques. The VQGAN learns a codebook of context-rich visual parts, whose composition is then modeled with an autoregressive transformer.
The codebook is generated through a process called vector quantization (VQ), i.e., the “VQ” part of “VQGAN.” Vector quantization is a signal processing technique for encoding vectors. It represents all visual parts found in the convolutional step in a quantized form, making it less computationally expensive once passed to a transformer network.
One can think of vector quantization as a process of dividing vectors into groups that have approximately the same number of points closest to them. Each group is then represented by a centroid (codeword), usually obtained via k-means or any other clustering algorithm. In the end, one learns a dictionary of centroids (codebook) and their corresponding members.
Now a brief about CLIP:
CLIP( Contrastive Language–Image Pre-training ) a model trained to determine which caption from a set of captions best fits with a given image.
OpenAI’s CLIP model aims to learn generic visual concept with natural language supervision. This is because sandard computer vision model only work well on specific task, and require significant effort to adapt to a new task, hence have weak generalization capabilities. CLIP bridges the gap via learning directly from raw text about images at a web scale level. CLIP does not directly optimize for the performance of a benchmark task (e.g. CIFAR), so as to keep its “zero-shot” capabilities for generalization. More interestingly, CLIP shows that scaling a simple pre-training task — which is to learn “which text matches with which image”, is sufficient to achieve competitive zero-shot performance on many image classification datasets.
CLIP trains a text encoder (Bag-of-Words or Text Transformer) and an image encoder (ResNet or Image Transformer) which learns feature representations of a given pair of text and image. The scaled cosine similarity matrix of the image and text feature is computed, and the diagonal values are minimized to force the image feature match its corresponding text feature.
VQGAN+CLIP:
CLIP guides VQGAN towards an image that is the best match to a given text. CLIP is the “Perceptor” and VQGAN is the “Generator”. VQGAN like all GANs VQGAN takes in a noise vector, and outputs a (realistic) image. CLIP on the other hand takes in an image and text, and outputs the image features and text features respectively. The similarity between image and text can be represented by the cosine similarity of the learnt feature vectors.
By leveraging CLIPs capacities as a “steering wheel”, we can use CLIP to guide a search through VQGAN’s latent space to find images that match a text prompt very well according to CLIP.
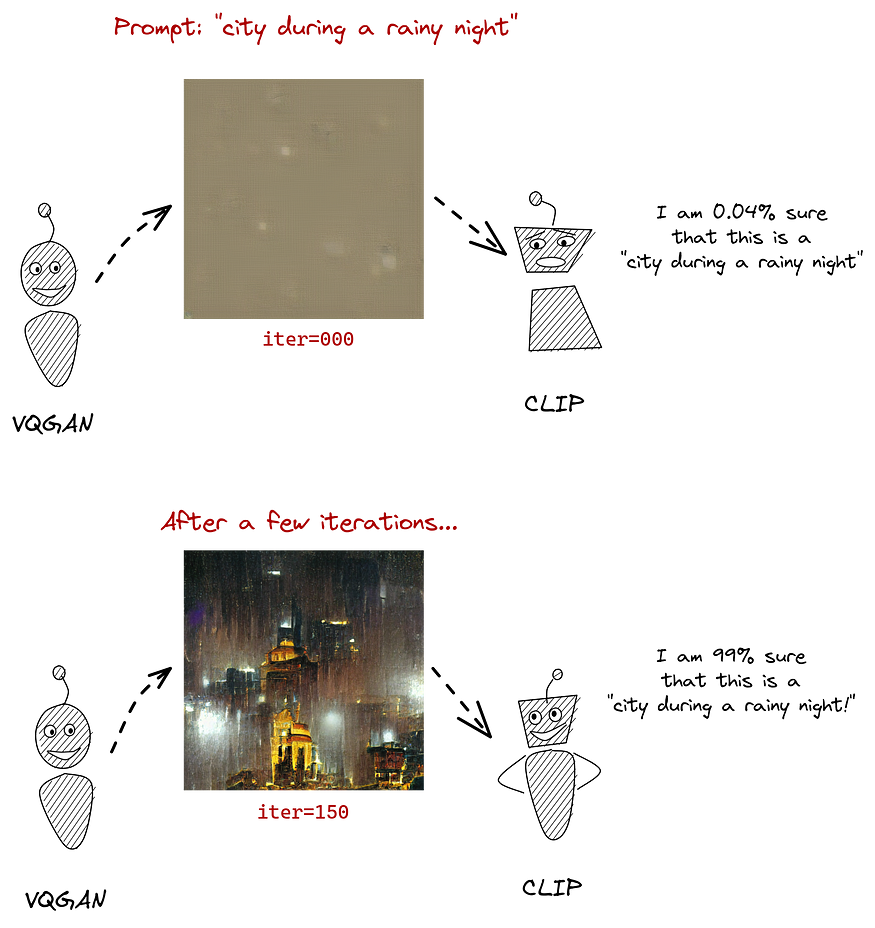
Now the fun part CODE:
##Install the dependencies
!pip install --user torch==1.9.0 torchvision==0.10.0 torchaudio==0.9.0 torchtext==0.10.0
!git clone https://github.com/openai/CLIP
!pip install taming-transformers
!git clone https://github.com/CompVis/taming-transformers.git
!pip install ftfy regex tqdm omegaconf pytorch-lightning
!pip install kornia
!pip install imageio-ffmpeg
!pip install einops
!mkdir steps
Import the libraries:
!pip install setuptools==59.5.0
import os
import torch
torch.hub.download_url_to_file('https://heibox.uni-heidelberg.de/d/a7530b09fed84f80a887/files/?p=%2Fconfigs%2Fmodel.yaml&dl=1',
'vqgan_imagenet_f16_16384.yaml')
torch.hub.download_url_to_file('https://heibox.uni-heidelberg.de/d/a7530b09fed84f80a887/files/?p=%2Fckpts%2Flast.ckpt&dl=1',
'vqgan_imagenet_f16_16384.ckpt')
import argparse
import math
from pathlib import Path
import sys
sys.path.insert(1, './taming-transformers')
# from IPython import display
from base64 import b64encode
from omegaconf import OmegaConf
from PIL import Image
import matplotlib.pyplot as plt
from taming.models import cond_transformer, vqgan
import taming.modules
from torch import nn, optim
from torch.nn import functional as F
from torchvision import transforms
from torchvision.transforms import functional as TF
from tqdm.notebook import tqdm
from CLIP import clip
import kornia.augmentation as K
import numpy as np
import imageio
from PIL import ImageFile, Image
from urllib.request import urlopen
ImageFile.LOAD_TRUNCATED_IMAGES = True
from pynvml.smi import nvmlInit, nvmlDeviceGetHandleByIndex, nvmlDeviceGetUtilizationRates
nvmlInit()
handle = nvmlDeviceGetHandleByIndex(0)
import warnings
warnings.filterwarnings("ignore")
IF you run with an error, restart the kernel, this is due to installing new packages requires a kernel restart
#Download sample photos
torch.hub.download_url_to_file('https://images.pexels.com/photos/3617500/pexels-photo-3617500.jpeg',
'aurora.jpeg')
torch.hub.download_url_to_file('https://images.pexels.com/photos/1660990/pexels-photo-1660990.jpeg',
'iceland_road.jpeg')
Build and Define the Functions.
def sinc(x):
return torch.where(x != 0, torch.sin(math.pi * x) / (math.pi * x), x.new_ones([]))
def lanczos(x, a):
cond = torch.logical_and(-a < x, x < a)
out = torch.where(cond, sinc(x) * sinc(x/a), x.new_zeros([]))
return out / out.sum()
def ramp(ratio, width):
n = math.ceil(width / ratio + 1)
out = torch.empty([n])
cur = 0
for i in range(out.shape[0]):
out[i] = cur
cur += ratio
return torch.cat([-out[1:].flip([0]), out])[1:-1]
def resample(input, size, align_corners=True):
n, c, h, w = input.shape
dh, dw = size
input = input.view([n * c, 1, h, w])
if dh < h:
kernel_h = lanczos(ramp(dh / h, 2), 2).to(input.device, input.dtype)
pad_h = (kernel_h.shape[0] - 1) // 2
input = F.pad(input, (0, 0, pad_h, pad_h), 'reflect')
input = F.conv2d(input, kernel_h[None, None, :, None])
if dw < w:
kernel_w = lanczos(ramp(dw / w, 2), 2).to(input.device, input.dtype)
pad_w = (kernel_w.shape[0] - 1) // 2
input = F.pad(input, (pad_w, pad_w, 0, 0), 'reflect')
input = F.conv2d(input, kernel_w[None, None, None, :])
input = input.view([n, c, h, w])
return F.interpolate(input, size, mode='bicubic', align_corners=align_corners)class ReplaceGrad(torch.autograd.Function):
@staticmethod
def forward(ctx, x_forward, x_backward):
ctx.shape = x_backward.shape
return x_forward
@staticmethod
def backward(ctx, grad_in):
return None, grad_in.sum_to_size(ctx.shape)
replace_grad = ReplaceGrad.apply
class ClampWithGrad(torch.autograd.Function):
@staticmethod
def forward(ctx, input, min, max):
ctx.min = min
ctx.max = max
ctx.save_for_backward(input)
return input.clamp(min, max)
@staticmethod
def backward(ctx, grad_in):
input, = ctx.saved_tensors
return grad_in * (grad_in * (input - input.clamp(ctx.min, ctx.max)) >= 0), None, None
clamp_with_grad = ClampWithGrad.applydef vector_quantize(x, codebook):
d = x.pow(2).sum(dim=-1, keepdim=True) + codebook.pow(2).sum(dim=1) - 2 * x @ codebook.T
indices = d.argmin(-1)
x_q = F.one_hot(indices, codebook.shape[0]).to(d.dtype) @ codebook
return replace_grad(x_q, x)
class Prompt(nn.Module):
def __init__(self, embed, weight=1., stop=float('-inf')):
super().__init__()
self.register_buffer('embed', embed)
self.register_buffer('weight', torch.as_tensor(weight))
self.register_buffer('stop', torch.as_tensor(stop))
def forward(self, input):
input_normed = F.normalize(input.unsqueeze(1), dim=2)
embed_normed = F.normalize(self.embed.unsqueeze(0), dim=2)
dists = input_normed.sub(embed_normed).norm(dim=2).div(2).arcsin().pow(2).mul(2)
dists = dists * self.weight.sign()
return self.weight.abs() * replace_grad(dists, torch.maximum(dists, self.stop)).mean()
def parse_prompt(prompt):
vals = prompt.rsplit(':', 2)
vals = vals + ['', '1', '-inf'][len(vals):]
return vals[0], float(vals[1]), float(vals[2])class MakeCutouts(nn.Module):
def __init__(self, cut_size, cutn, cut_pow=1):
super().__init__()
self.cut_size = cut_size
self.cutn = cutn
self.cut_pow = cut_pow
self.augs = nn.Sequential(
K.RandomAffine(degrees=15, translate=0.1, p=0.7, padding_mode='border'),
K.RandomPerspective(0.7,p=0.7),
K.ColorJitter(hue=0.1, saturation=0.1, p=0.7),
K.RandomErasing((.1, .4), (.3, 1/.3), same_on_batch=True, p=0.7),
)
self.noise_fac = 0.1
self.av_pool = nn.AdaptiveAvgPool2d((self.cut_size, self.cut_size))
self.max_pool = nn.AdaptiveMaxPool2d((self.cut_size, self.cut_size))
def forward(self, input):
slideY, slideX = input.shape[2:4]
max_size = min(slideX, slideY)
min_size = min(slideX, slideY, self.cut_size)
cutouts = []
for _ in range(self.cutn):
cutout = (self.av_pool(input) + self.max_pool(input))/2
cutouts.append(cutout)
batch = self.augs(torch.cat(cutouts, dim=0))
if self.noise_fac:
facs = batch.new_empty([self.cutn, 1, 1, 1]).uniform_(0, self.noise_fac)
batch = batch + facs * torch.randn_like(batch)
return batchdef load_vqgan_model(config_path, checkpoint_path):
config = OmegaConf.load(config_path)
if config.model.target == 'taming.models.vqgan.VQModel':
model = vqgan.VQModel(**config.model.params)
model.eval().requires_grad_(False)
model.init_from_ckpt(checkpoint_path)
elif config.model.target == 'taming.models.vqgan.GumbelVQ':
model = vqgan.GumbelVQ(**config.model.params)
model.eval().requires_grad_(False)
model.init_from_ckpt(checkpoint_path)
elif config.model.target == 'taming.models.cond_transformer.Net2NetTransformer':
parent_model = cond_transformer.Net2NetTransformer(**config.model.params)
parent_model.eval().requires_grad_(False)
parent_model.init_from_ckpt(checkpoint_path)
model = parent_model.first_stage_model
else:
raise ValueError(f'unknown model type: {config.model.target}')
del model.loss
return modeldef resize_image(image, out_size):
ratio = image.size[0] / image.size[1]
area = min(image.size[0] * image.size[1], out_size[0] * out_size[1])
size = round((area * ratio)**0.5), round((area / ratio)**0.5)
return image.resize(size, Image.LANCZOS)
Download the models
model_name = "vqgan_imagenet_f16_16384"
images_interval = 50
width = 512
height = 512
init_image = ""
seed = 42
BASE_PATH = '../input/flickr-image-dataset/flickr30k_images/flickr30k_images/'
args = argparse.Namespace(
noise_prompt_seeds=[],
noise_prompt_weights=[],
size=[width, height],
init_image=init_image,
init_weight=0.,
clip_model='ViT-B/32',
vqgan_config=f'{model_name}.yaml',
vqgan_checkpoint=f'{model_name}.ckpt',
step_size=0.13,
cutn=32,
cut_pow=1.,
display_freq=images_interval,
seed=seed,
)
device = torch.device('cuda:0' if torch.cuda.is_available() else 'cpu')
print('Using device:', device)
model = load_vqgan_model(args.vqgan_config, args.vqgan_checkpoint).to(device)
perceptor = clip.load(args.clip_model, jit=False)[0].eval().requires_grad_(False).to(device)
The Main Function Definition
def inference(text,
seed,
step_size,
max_iterations,
width,
height,
init_image,
init_weight,
target_images,
cutn,
cut_pow,
video_file
):
all_frames = []
size=[width, height]
texts = text
init_weight=init_weight
if init_image:
init_image = init_image
else:
init_image = ""
if target_images:
target_images = target_images
else:
target_images = ""
max_iterations = max_iterations
model_names={"vqgan_imagenet_f16_16384": 'ImageNet 16384',
"vqgan_imagenet_f16_1024":"ImageNet 1024",
'vqgan_openimages_f16_8192':'OpenImages 8912',
"wikiart_1024":"WikiArt 1024",
"wikiart_16384":"WikiArt 16384",
"coco":"COCO-Stuff",
"faceshq":"FacesHQ",
"sflckr":"S-FLCKR"}
name_model = model_names[model_name]
if target_images == "None" or not target_images:
target_images = []
else:
target_images = target_images.split("|")
target_images = [image.strip() for image in target_images]
texts = [phrase.strip() for phrase in texts.split("|")]
if texts == ['']:
texts = []
if texts:
print('Using texts:', texts)
if target_images:
print('Using image prompts:', target_images)
if seed is None or seed == -1:
seed = torch.seed()
else:
seed = seed
torch.manual_seed(seed)
print('Using seed:', seed)
cut_size = perceptor.visual.input_resolution
f = 2**(model.decoder.num_resolutions - 1)
make_cutouts = MakeCutouts(cut_size, cutn, cut_pow=cut_pow)
toksX, toksY = size[0] // f, size[1] // f
sideX, sideY = toksX * f, toksY * f
if args.vqgan_checkpoint == 'vqgan_openimages_f16_8192.ckpt':
e_dim = 256
n_toks = model.quantize.n_embed
z_min = model.quantize.embed.weight.min(dim=0).values[None, :, None, None]
z_max = model.quantize.embed.weight.max(dim=0).values[None, :, None, None]
else:
e_dim = model.quantize.e_dim
n_toks = model.quantize.n_e
z_min = model.quantize.embedding.weight.min(dim=0).values[None, :, None, None]
z_max = model.quantize.embedding.weight.max(dim=0).values[None, :, None, None]
if init_image:
if 'http' in init_image:
img = Image.open(urlopen(init_image))
else:
img = Image.open(init_image)
pil_image = img.convert('RGB')
pil_image = pil_image.resize((sideX, sideY), Image.LANCZOS)
pil_tensor = TF.to_tensor(pil_image)
z, *_ = model.encode(pil_tensor.to(device).unsqueeze(0) * 2 - 1)
else:
one_hot = F.one_hot(torch.randint(n_toks, [toksY * toksX], device=device), n_toks).float()
# z = one_hot @ model.quantize.embedding.weight
if args.vqgan_checkpoint == 'vqgan_openimages_f16_8192.ckpt':
z = one_hot @ model.quantize.embed.weight
else:
z = one_hot @ model.quantize.embedding.weight
z = z.view([-1, toksY, toksX, e_dim]).permute(0, 3, 1, 2)
z = torch.rand_like(z)*2
z_orig = z.clone()
z.requires_grad_(True)
opt = optim.Adam([z], lr=step_size)
normalize = transforms.Normalize(mean=[0.48145466, 0.4578275, 0.40821073],
std=[0.26862954, 0.26130258, 0.27577711])
pMs = []
for prompt in texts:
txt, weight, stop = parse_prompt(prompt)
embed = perceptor.encode_text(clip.tokenize(txt).to(device)).float()
pMs.append(Prompt(embed, weight, stop).to(device))
for prompt in target_images:
path, weight, stop = parse_prompt(prompt)
img = Image.open(path)
pil_image = img.convert('RGB')
img = resize_image(pil_image, (sideX, sideY))
batch = make_cutouts(TF.to_tensor(img).unsqueeze(0).to(device))
embed = perceptor.encode_image(normalize(batch)).float()
pMs.append(Prompt(embed, weight, stop).to(device))
for seed, weight in zip(args.noise_prompt_seeds, args.noise_prompt_weights):
gen = torch.Generator().manual_seed(seed)
embed = torch.empty([1, perceptor.visual.output_dim]).normal_(generator=gen)
pMs.append(Prompt(embed, weight).to(device))
def synth(z):
if args.vqgan_checkpoint == 'vqgan_openimages_f16_8192.ckpt':
z_q = vector_quantize(z.movedim(1, 3), model.quantize.embed.weight).movedim(3, 1)
else:
z_q = vector_quantize(z.movedim(1, 3), model.quantize.embedding.weight).movedim(3, 1)
return clamp_with_grad(model.decode(z_q).add(1).div(2), 0, 1)
@torch.no_grad()
def checkin(i, losses):
losses_str = ', '.join(f'{loss.item():g}' for loss in losses)
tqdm.write(f'i: {i}, loss: {sum(losses).item():g}, losses: {losses_str}')
out = synth(z)
# TF.to_pil_image(out[0].cpu()).save('progress.png')
# display.display(display.Image('progress.png'))
res = nvmlDeviceGetUtilizationRates(handle)
print(f'gpu: {res.gpu}%, gpu-mem: {res.memory}%')
def ascend_txt():
# global i
out = synth(z)
iii = perceptor.encode_image(normalize(make_cutouts(out))).float()
result = []
if init_weight:
result.append(F.mse_loss(z, z_orig) * init_weight / 2)
#result.append(F.mse_loss(z, torch.zeros_like(z_orig)) * ((1/torch.tensor(i*2 + 1))*init_weight) / 2)
for prompt in pMs:
result.append(prompt(iii))
img = np.array(out.mul(255).clamp(0, 255)[0].cpu().detach().numpy().astype(np.uint8))[:,:,:]
img = np.transpose(img, (1, 2, 0))
# imageio.imwrite('./steps/' + str(i) + '.png', np.array(img))
img = Image.fromarray(img).convert('RGB')
all_frames.append(img)
return result, np.array(img)
def train(i):
opt.zero_grad()
lossAll, image = ascend_txt()
if i % args.display_freq == 0:
checkin(i, lossAll)
loss = sum(lossAll)
loss.backward()
opt.step()
with torch.no_grad():
z.copy_(z.maximum(z_min).minimum(z_max))
return image
i = 0
try:
with tqdm() as pbar:
while True:
image = train(i)
if i == max_iterations:
break
i += 1
pbar.update()
except KeyboardInterrupt:
pass
writer = imageio.get_writer(video_file + '.mp4', fps=20)
for im in all_frames:
writer.append_data(np.array(im))
writer.close()
# all_frames[0].save('out.gif',
# save_all=True, append_images=all_frames[1:], optimize=False, duration=80, loop=0)
return imagedef load_image( infilename ) :
img = Image.open( infilename )
img.load()
data = np.asarray( img, dtype="int32" )
return datadef display_result(img) :
plt.figure(figsize=(9,9))
plt.imshow(img)
plt.axis('off')
Test Run 1:
img = inference(
text = 'underwater city ',
seed = 2,
step_size = 0.12,
max_iterations = 300,
width = 512,
height = 512,
init_image = '',
init_weight = 0.004,
target_images = '',
cutn = 64,
cut_pow = 0.3,
video_file = "test1"
)
display_result(img)
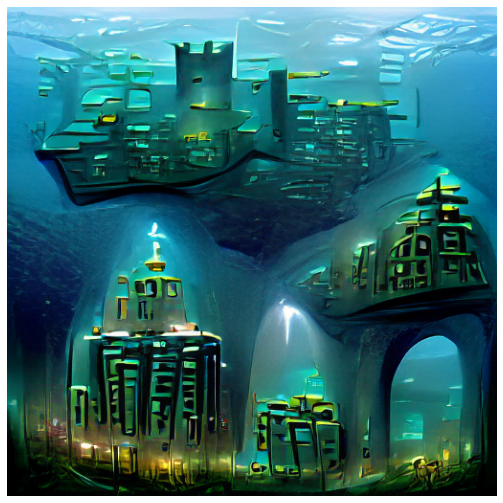
#Download the output in video format
from IPython.display import HTML
from base64 import b64encode
mp4 = open('test1.mp4','rb').read()
data_url = "data:video/mp4;base64," + b64encode(mp4).decode()
HTML("""
<video width=500 loop="true" autoplay="autoplay" controls muted>
<source src="%s" type="video/mp4">
</video>
""" % data_url)
Test Run 2
img = inference(
text = 'winter in train',
seed = 191,
step_size = 0.13,
max_iterations = 700,
width = 512,
height = 512,
init_image = '',
init_weight = 0.0,
target_images = '',
cutn = 64,
cut_pow = 1.0,
video_file = "winter_in_train"
)
display_result(img)
mp4 = open('winter_in_train.mp4','rb').read()
data_url = "data:video/mp4;base64," + b64encode(mp4).decode()
HTML("""
<video width=500 loop="true" autoplay="autoplay" controls muted>
<source src="%s" type="video/mp4">
</video>
""" % data_url)
Test Run 3:
img = inference(
text = 'mutation tree and flower',
seed = 79472135470,
step_size = 0.12,
max_iterations = 300,
width = 512,
height = 512,
init_image = '',
init_weight = 0.024,
target_images = '',
cutn = 32,
cut_pow = 1.0,
video_file = "mutation"
)
display_result(img)
mp4 = open('mutation.mp4','rb').read()
data_url = "data:video/mp4;base64," + b64encode(mp4).decode()
HTML("""
<video width=500 loop="true" autoplay="autoplay" controls muted>
<source src="%s" type="video/mp4">
</video>
""" % data_url)
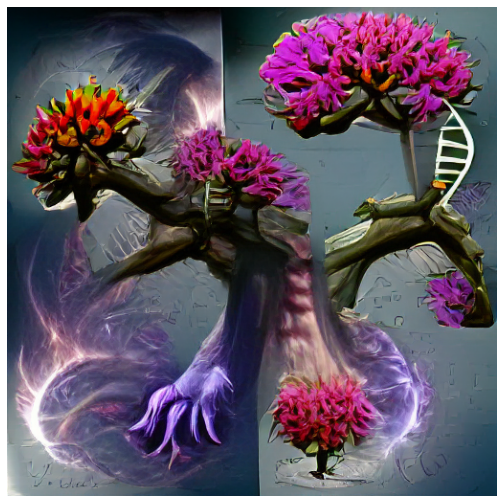
Test Run 4
img = inference(
text = 'Angels of the Universe',
seed = 1011,
step_size = 0.12,
max_iterations = 700,
width = 512,
height = 512,
init_image = '',
init_weight = 0.0,
target_images = '',
cutn = 32,
cut_pow = 1.0,
video_file = "angels_of_the_universe"
)
display_result(img)
mp4 = open('angels_of_the_universe.mp4','rb').read()
data_url = "data:video/mp4;base64," + b64encode(mp4).decode()
HTML("""
<video width=500 loop="true" autoplay="autoplay" controls muted>
<source src="%s" type="video/mp4">
</video>
""" % data_url)
Test Run 5 ~ with init_image
img = inference(
text = 'Fireflies in the Garden',
seed = 201,
step_size = 0.12,
max_iterations = 400,
width = 512,
height = 512,
init_image = 'https://i1.sndcdn.com/artworks-3TjRVLDyziCxsPFm-eaTwZw-t500x500.jpg',
init_weight = 0.0,
target_images = '',
cutn = 64,
cut_pow = 1.0,
video_file = "fireflies"
)
display_result(img)mp4 = open('fireflies.mp4','rb').read()
data_url = "data:video/mp4;base64," + b64encode(mp4).decode()
HTML("""
<video width=500 loop="true" autoplay="autoplay" controls muted>
<source src="%s" type="video/mp4">
</video>
""" % data_url)
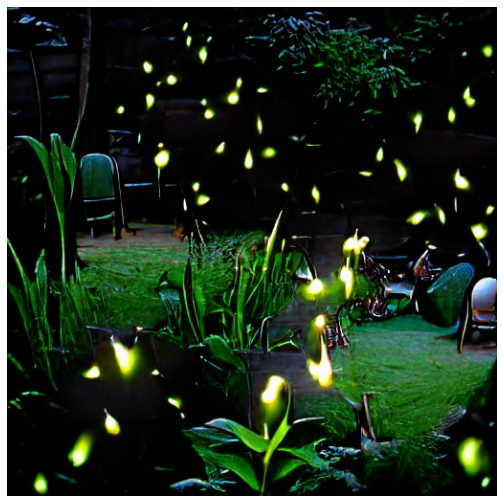
Try out different combinations of keywords
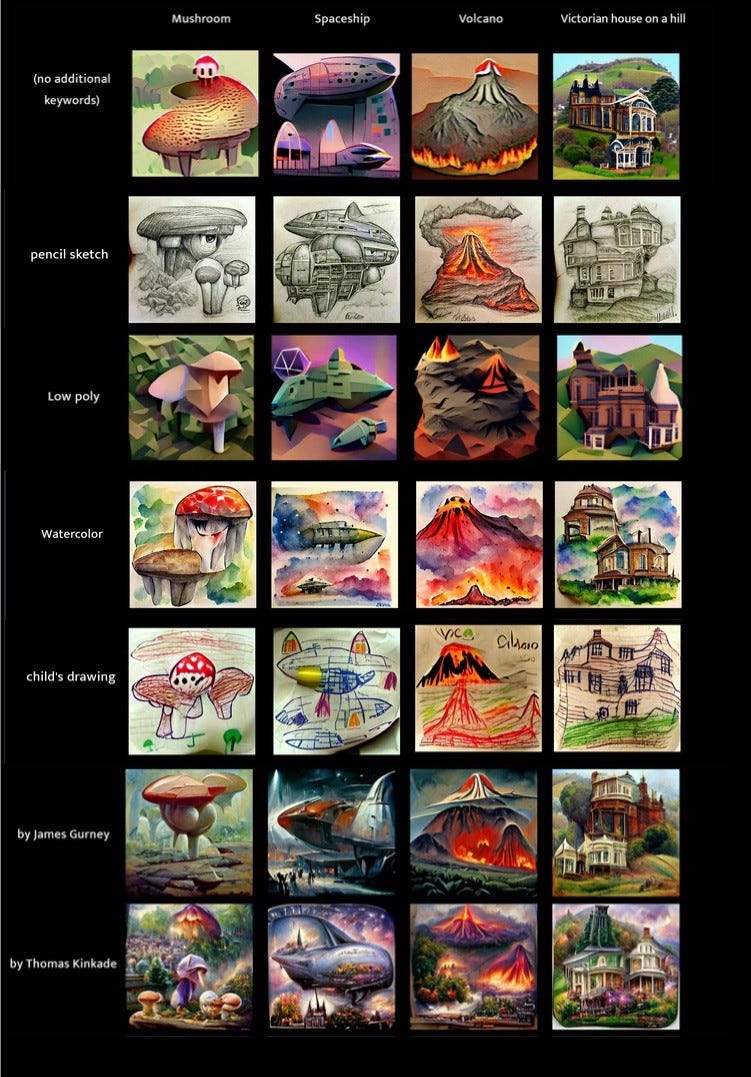
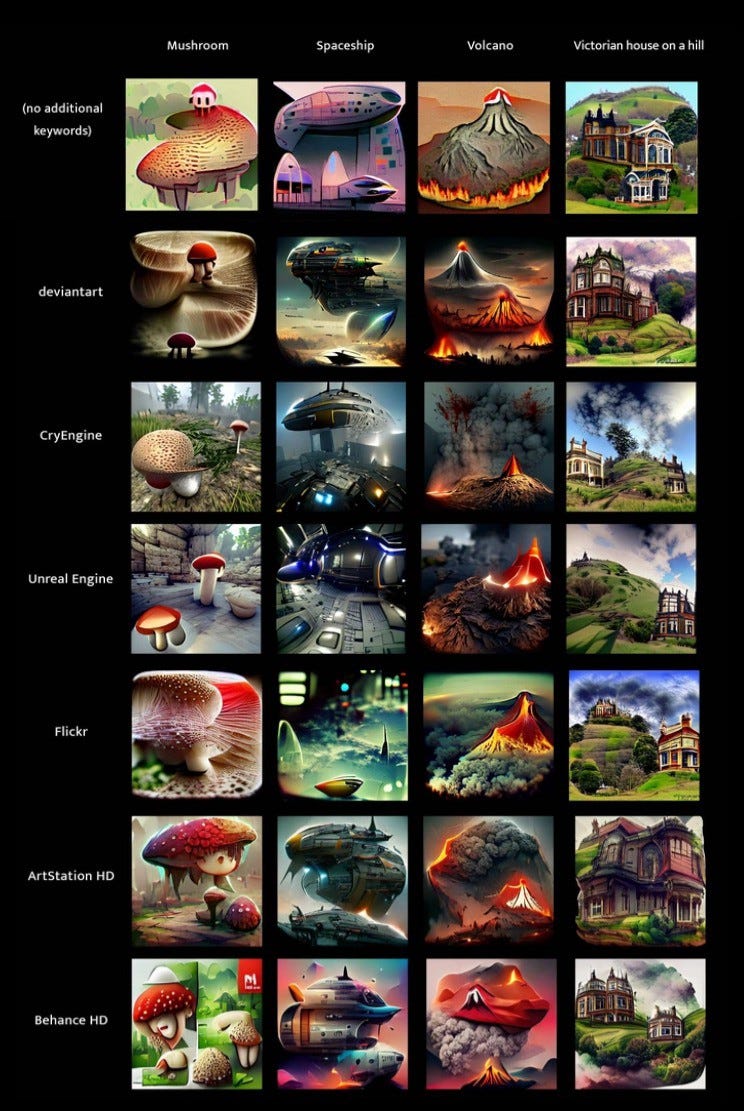
References
- VQGAN_CLIP( hHugging Face )
- The Illustrated VQGAN
- VQGAN+CLIP — How does it work?
- Image Generation Based on Abstract Concepts Using CLIP + BigGAN
Well, that's it. i know its a long list of scripts but don't worry i got that covered too, just visit my github to download the repo or Kaggle to see the implementation in action.
GitHub Repo: https://github.com/rupak-roy/VQGAN-CLIP
Kaggle Implementation: https://www.kaggle.com/code/rupakroy/text-to-art-vqgan-clip-updated
Thanks again, for your time, if you enjoyed this short article there are tons of topics in advanced analytics, data science, and machine learning available in my medium repo. https://medium.com/@bobrupakroy
Some of my alternative internet presences are Facebook, Instagram, Udemy, Blogger, Issuu, Slideshare, Scribd, and more.
Also available on Quora @ https://www.quora.com/profile/Rupak-Bob-Roy
Let me know if you need anything. Talk Soon.
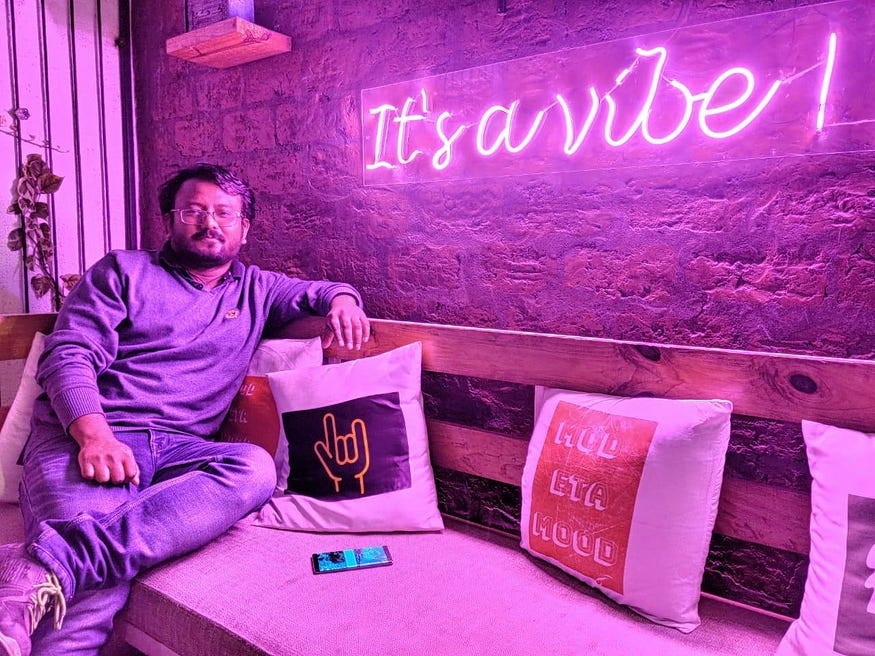
Comments
Post a Comment