Halving GridSearch The next best alternative to the exhaustive cousins Random & GridSearch
Halving GridSearch
The next best alternative to the exhaustive cousins Random & GridSearch
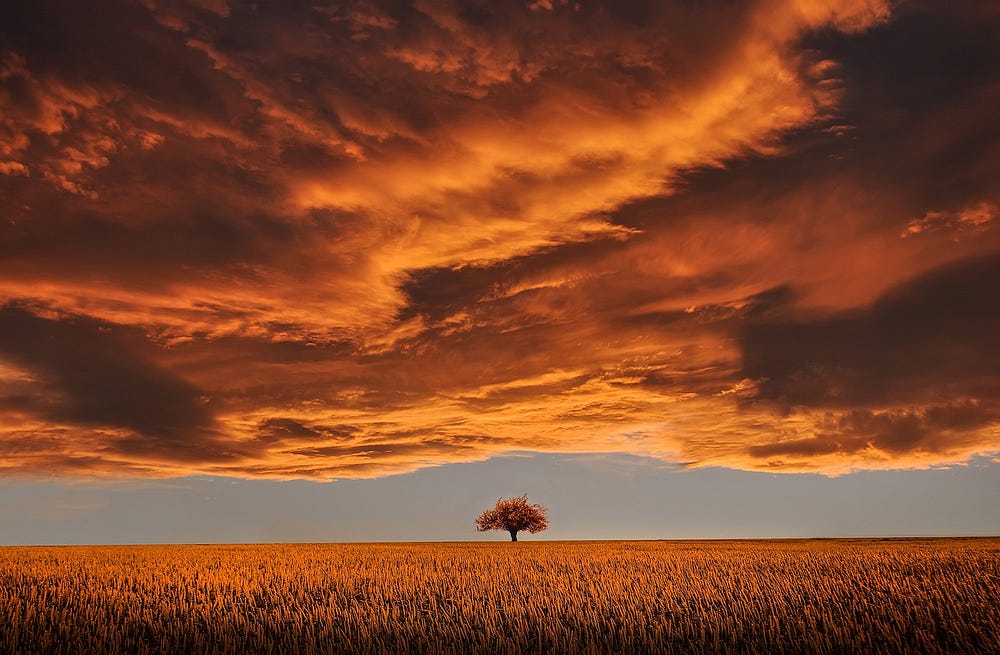
hey there, how things are holding? i hope its all fine. Hey today i bring you something new and very useful indeed called Halving Grid Search for hyperparameters tuning.
Halving Grid Search, a new class of successive Halving, where training is performed on the subsets of data, rather than on all the data. The worst performing data are filtered out by training them on a small subset of data. After N number iterations select the best data/candidates leading to a faster evaluation time.
In General, the Halving GridSearch performs 11x times faster than our regular GridSearch.
According to sklearn,
HalvingGridSearch: The search strategy starts evaluating all the candidates with a small amount of resources and iteratively selects the best candidates, using more and more resources.
Parameter list:~
class sklearn.model_selection.HalvingGridSearchCV(estimator, param_grid, *, factor=3, resource='n_samples', max_resources='auto', min_resources='exhaust', aggressive_elimination=False, cv=5, scoring=None, refit=True, error_score=nan, return_train_score=True, random_state=None,
n_jobs=None, verbose=0)
Let’s understand on how to apply Halving GridSEarch with a sample.
from sklearn.datasets import load_iris
from sklearn.ensemble import RandomForestClassifier
from sklearn.experimental import enable_halving_search_cv # noqa
from sklearn.model_selection import HalvingGridSearchCV
X, y = load_iris(return_X_y=True)
clf = RandomForestClassifier(random_state=0)
param_grid = {"max_depth": [3, None],"min_samples_split": [5, 10]}
search = HalvingGridSearchCV(clf, param_grid, resource='n_estimators',max_resources=10,random_state=0).fit(X, y)
search.best_params_
Out[9]: {‘max_depth’: None, ‘min_samples_split’: 5, ‘n_estimators’: 9}
Here we are the output, the optimized hyperparameter.
Let’s also explore another variant of Halving GridSearch
HalvingRandomSearchCV
“The candidates are sampled at random from the parameter space and the number of sampled candidates is determined by n_candidates.”
from sklearn.datasets import load_iris
from sklearn.ensemble import RandomForestClassifier
from sklearn.experimental import enable_halving_search_cv # noqa
from sklearn.model_selection import HalvingRandomSearchCV
from scipy.stats import randint
import numpy as np
X, y = load_iris(return_X_y=True)
clf = RandomForestClassifier(random_state=0)
np.random.seed(0)
param_distributions = {"max_depth": [3, None],
"min_samples_split": randint(2, 11)}
search = HalvingRandomSearchCV(clf, param_distributions,
resource='n_estimators',
max_resources=10,
random_state=0).fit(X, y)
search.best_params_
Out[10]: {‘max_depth’: 3, ‘min_samples_split’: 3, ‘n_estimators’: 9}
So that's it…………i hope you enjoyed this short and useful article.
Next, we will look into PowerTransformers to handle highly skewed data.
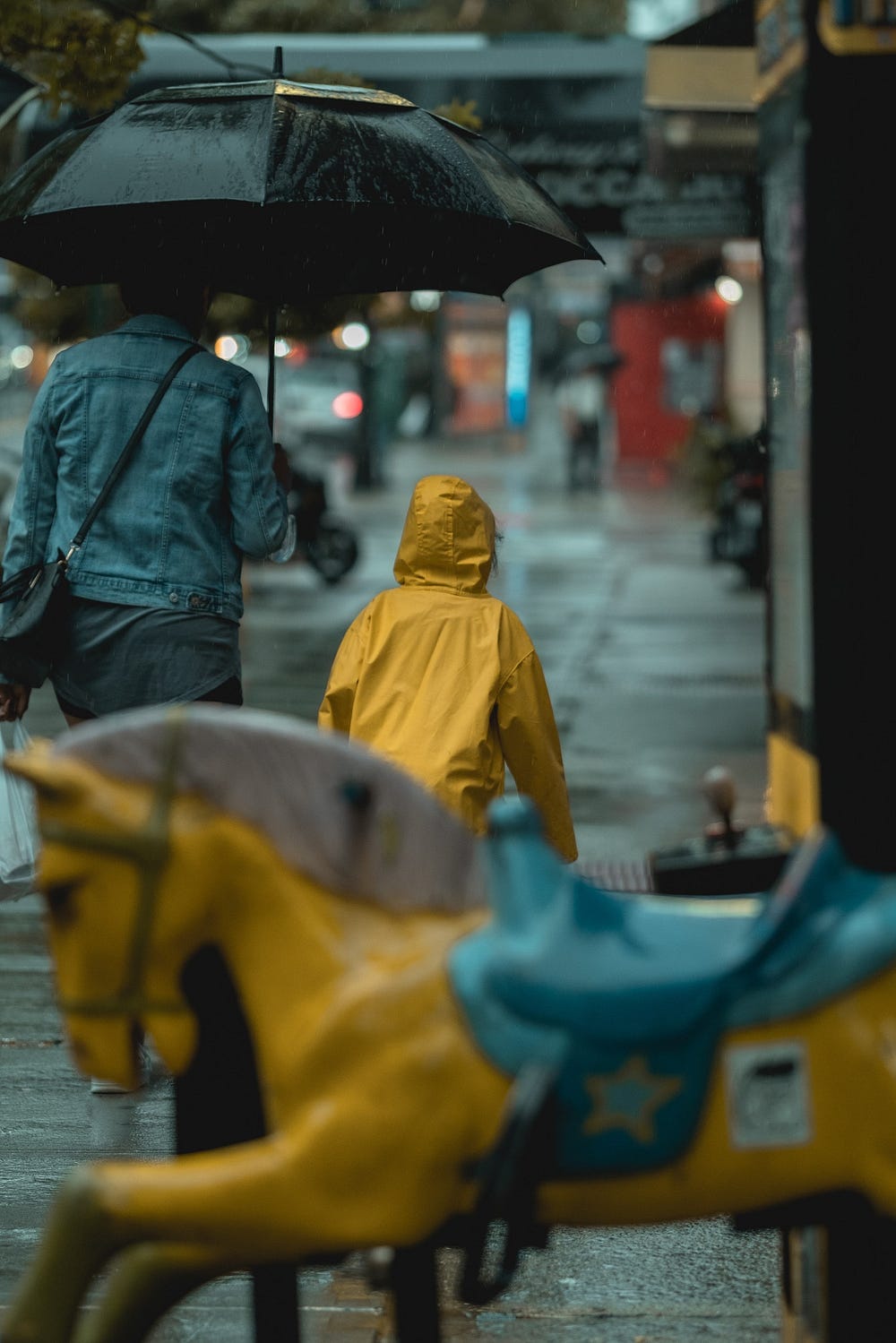
Thanks again, for your time, if you enjoyed this short article there are tons of topics in advanced analytics, data science, and machine learning available in my medium repo. https://medium.com/@bobrupakroy
Some of my alternative internet presences Facebook, Instagram, Udemy, Blogger, Issuu, Slideshare, Scribd and more.
Also available on Quora @ https://www.quora.com/profile/Rupak-Bob-Roy
Let me know if you need anything. Talk Soon.
Comments
Post a Comment