Synthetic Data Vault. A deep learning approach for building synthetic data
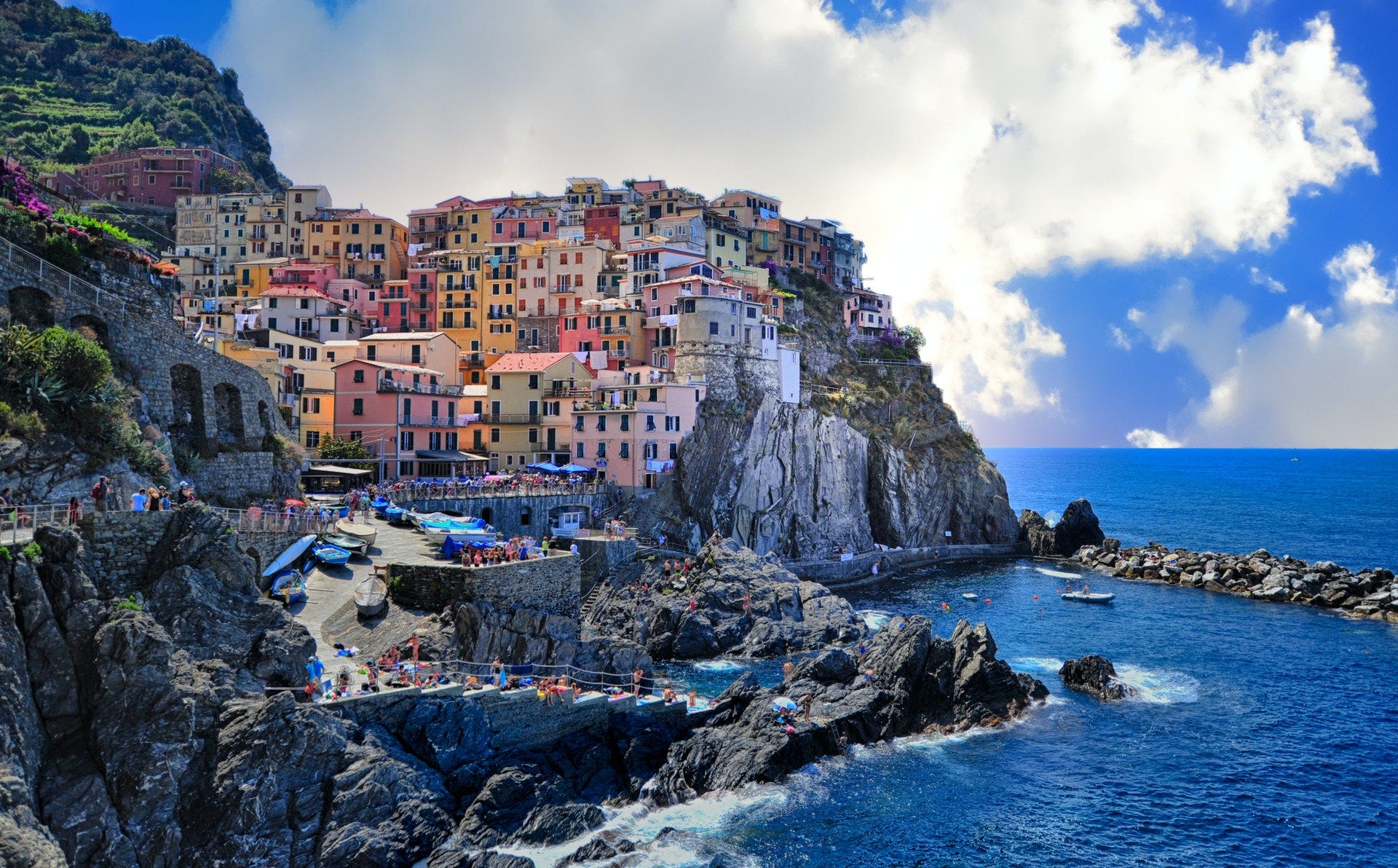
Hey hi hellooooo how are you, welcome to another chapter of building synthetic data. In our previous chapters/articles, we have seen numerous ways to generate or synthetic data like smote, smote-nc, oss, once, enn, CNN, ensemble approach, and much more. Now this time we will take a deep learning approach.
The Deep Learning approach also has a few variants, we will try to go through each short and simple.
- ) GaussianCopula: in mathematics, a copula is distributed over the unit cube[0,1] which is constructed from a multivariate normal distribution over Rd by using the probability integral transform. Intuitively a coupla is a mathematical function that allows us to describe the joint distribution of multiple random variables by analyzing the dependencies between their marginal distributions. Still, confused? don't worry at the end of the article i will give you the repo for detailed documentation.
Now, let’s see how can we apply this using python
#first we need to install the package
pip install sdv
#load a sample dataset
from sdv.demo import load_tabular_demo
data = load_tabular_demo('student_placements')
data.head()
Let us use the GaussianCopula to learn the sample data and then generate a synthetic dataset capturing the characteristics of the sample dataset above.
from sdv.tabular import GaussianCopula
model = GaussianCopula()
model.fit(data)
Now to generate new synthetic data all we need to do is call the sample.method from the model passing the number of rows that we want to generate
new_data = model.sample(200)
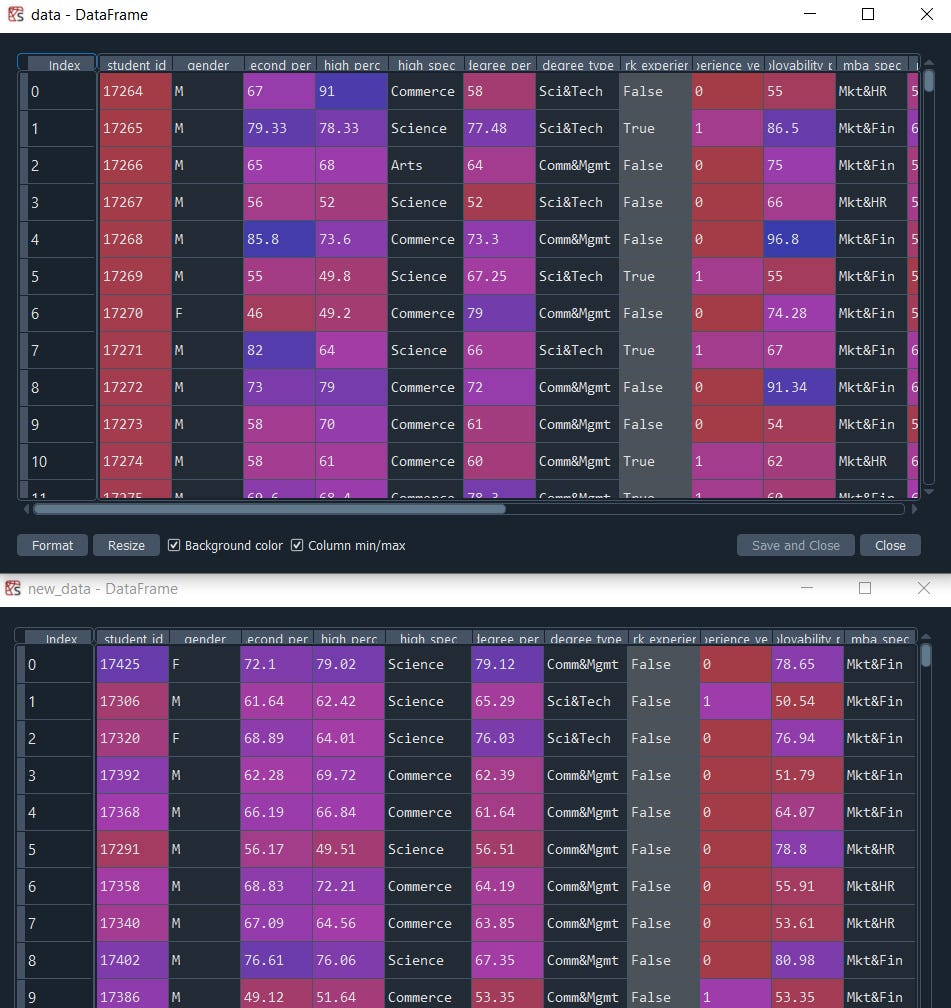
We can observe identical data to the original table. Finally, save and share the model will be a convenient to generate a synthetic version of your data directly in systems that do not have access to the original data source
model.save('my_model.pkl')
Load back the model with GaussianCopula load and start generating new sample data identical to the original one/
loaded = GaussianCopula.load('my_model.pkl')
new_data = loaded.sample(200)
One of the important things u might have missed if you have checked the student_id column which needs to be unique and in our synthetic data we have generated some values more than once.
The way to fix this is to define the unique value column as a ‘primary key’ in our model.
model = GaussianCopula(primary_key='student_id')
model.fit(data)
new_data = model.sample(200)
Anonymizing Personally Identifiable Information (PII)
There are some situations where the data will contain personally identifiable information which cannot disclose. Thus we will replace the information with simulated data that looks similar to the real one but doesn't contain the original values.
data_pii = load_tabular_demo('student_placements_pii')
data_pii.head()
model = GaussianCopula(primary_key='student_id',anonymize_fields={'address': 'address' })
model.fit(data_pii)
new_data_pii = model.sample(200)
new_data_pii.head()
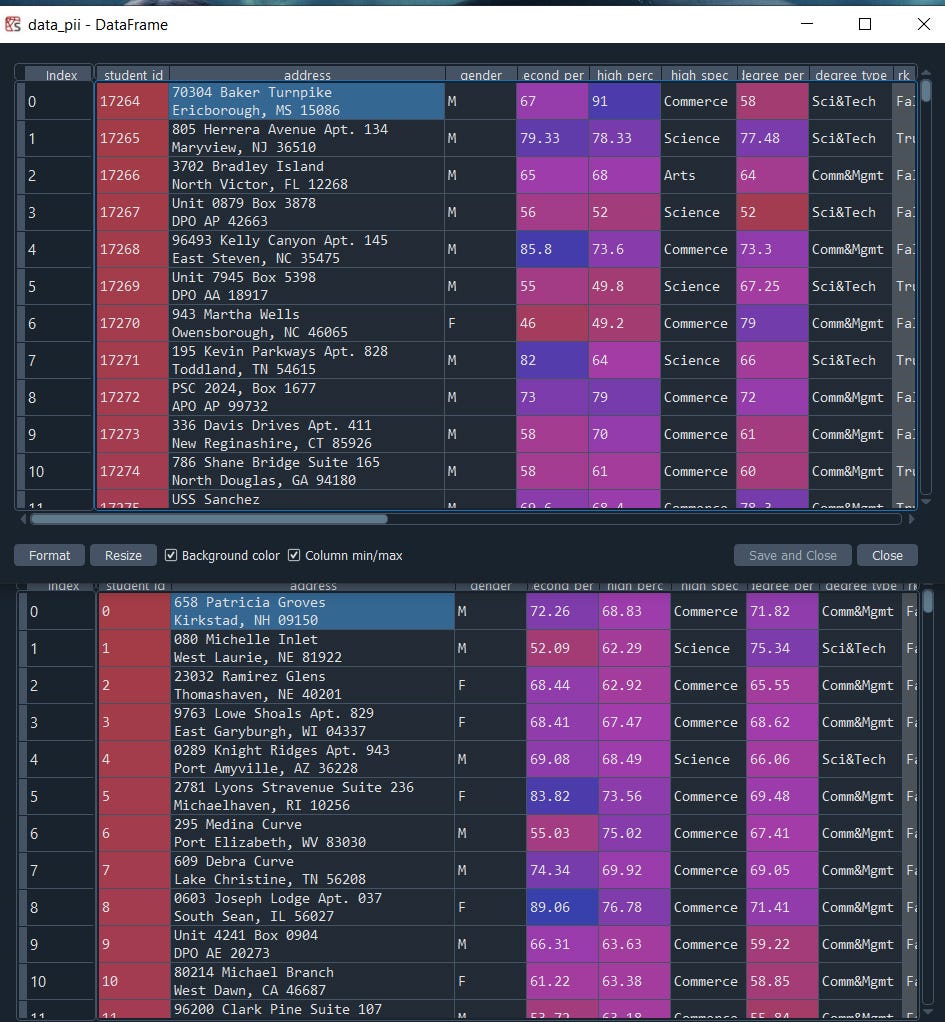
That's it, just use one parameter anonymize_fields we can clearly see the difference.
I hope you enjoyed it,
Likewise, I will try my best to bring more new ways of Data Science.
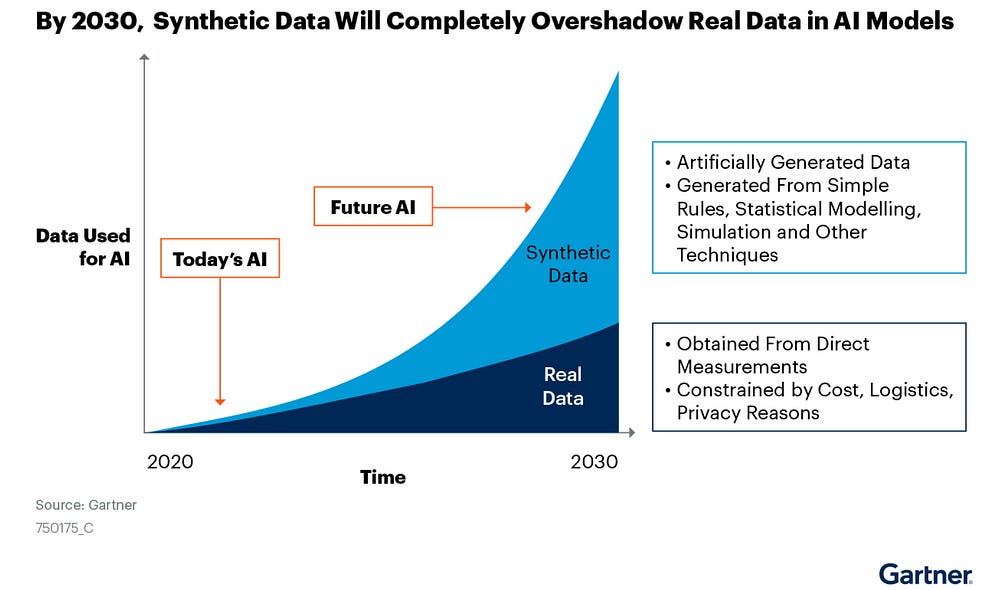
Read the article:
https://blogs.nvidia.com/blog/2021/06/08/what-is-synthetic-data/
Next, we have a Conditional GAN- CTGAN, Generative Adversarial Network-based Deep Learning data synthesizer which was presented at the NeurIPS 2020. sounds interesting? see ya in the next article.
Some of my alternative internet presences Facebook, Instagram, Udemy, Blogger, Issuu, and more.
Also available on Quora @ https://www.quora.com/profile/Rupak-Bob-Roy
Have a good day.
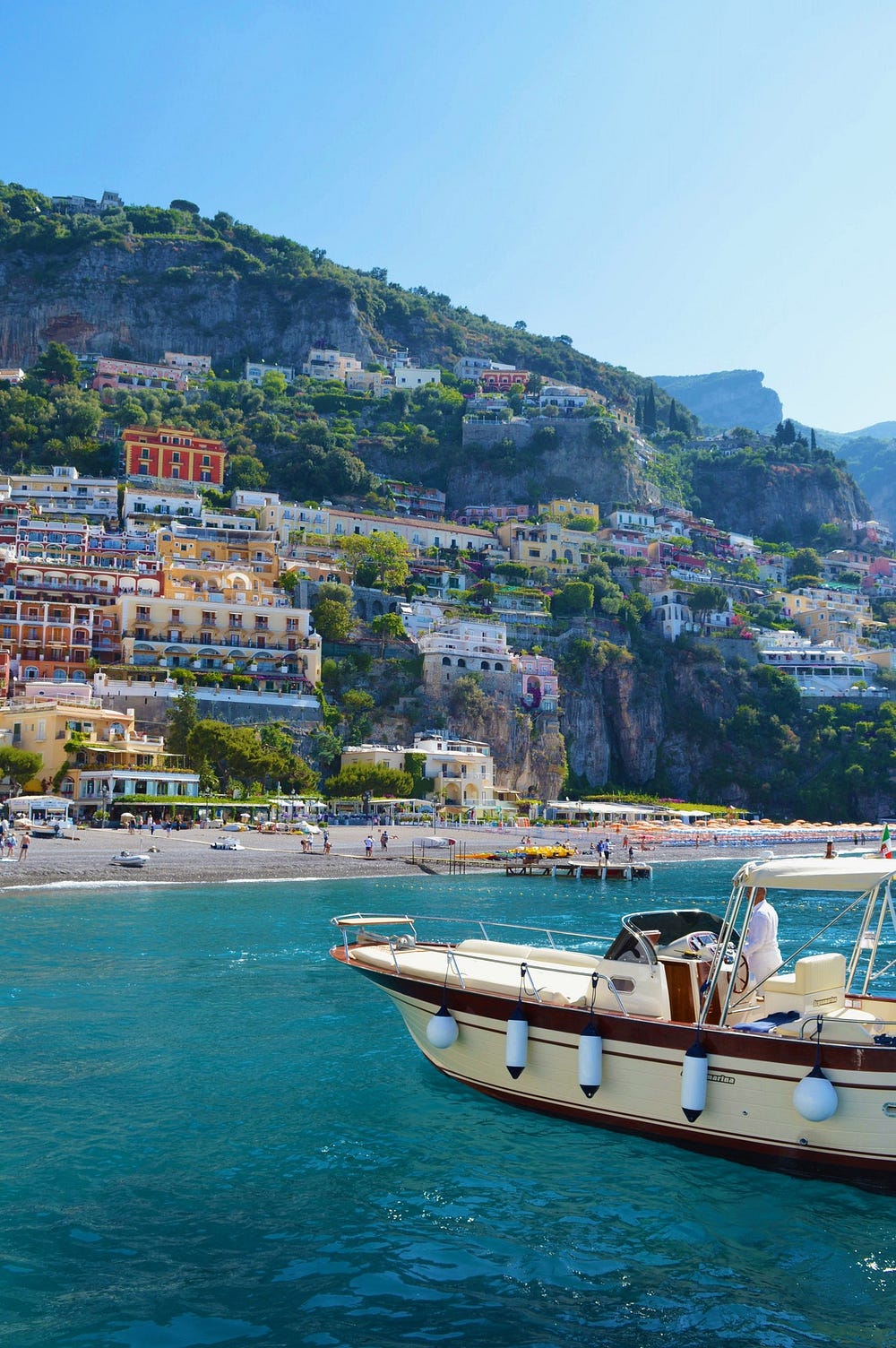
Comments
Post a Comment