OneClassSVM. A Density Based Outlier / Anomaly Detection
One-Class SVM sounds interesting? SVM as we know The support vector machine, algorithm developed mainly for non-linear data classification tasks.
The modification of the algorithm that captures the density of the majority class is referred to as One-Class SVM
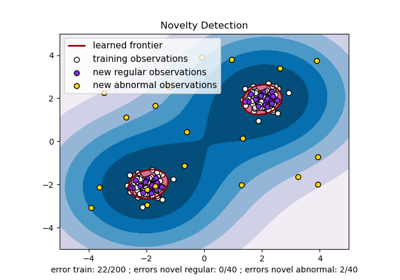
Let’s see how can we perform the One-ClassSVM
import numpy as np
import pandas as pd
from sklearn.model_selection import train_test_split
from sklearn.metrics import mean_absolute_error
df = pd.read_csv("housing.csv")
data = df.values
#define the X and y
X, y = data[:, :-1], data[:, -1]
# split into train and test sets
X_train, X_test, y_train, y_test = train_test_split(X, y, test_size=0.33, random_state=1)
Load the dataset, define X and y then split into Train and Test
from sklearn.svm import OneClassSVM
# identify outliers in the training dataset
ocs = OneClassSVM(nu=0.01)
yhat = ocs.fit_predict(X_train)
# select all rows that are not outliers
mask = yhat != -1
X_train, y_train = X_train[mask, :], y_train[mask]
#Else impute the outliers with nan then replace with mean or median
#mask = yhat ==-1
#Impute with nan
#-----replace with mean or median----
#The class provides the “nu” argument that specifies a approx ratio of outliers in the dataset, default = 0.1
The full list of One-Class SVM parameters
OneClassSVM(*, self, kernel='rbf', degree=3, gamma='scale', coef0=0.0, tol=1e-3, nu=0.5, shrinking=True, cache_size=200, verbose=False, max_iter=-1)
Now we can carry forward our cleaned dataset for any modeling tasks.
from sklearn.model_selection import KFold, cross_val_score
kfold =KFold(n_splits=3)
#no. of base classifiers
num_trees = 500
from sklearn.tree import DecisionTreeRegressor
from sklearn.ensemble import BaggingRegressor
#bagging classifier
model_regressor = BaggingRegressor(base_estimator = DecisionTreeRegressor(),n_estimators=num_trees,random_state=3)
results1 =cross_val_score(model_regressor,X_train,y_train,cv = kfold)
results1.mean()
model_regressor.fit(X_train,y_train)
model_regressor.predict(X_test)
# evaluate the model
yhat = model_regressor.predict(X_test)
# evaluate predictions
mae = mean_absolute_error(y_test, yhat)
print('MAE: %.3f' % mae)
Well, i have added 2 accuracy checkpoints… K-fold Cross_validation_score and Mean Absolute Error (MAE)
Done….!
Do Remember OneClassSVM or any other OUTLIER Treatment is performed on Train ‘X’ dataset that is excluding of target ‘y’ dataset.
Next, we have another interesting outlier treatment algorithm called “LocalOutlierFactor” interested? keep in touch
See you soon…..
Some of my alternative internet presences are Facebook, Instagram, Udemy, Blogger, Issuu, and more.
Also available on Quora @ https://www.quora.com/profile/Rupak-Bob-Roy
Have a good day.
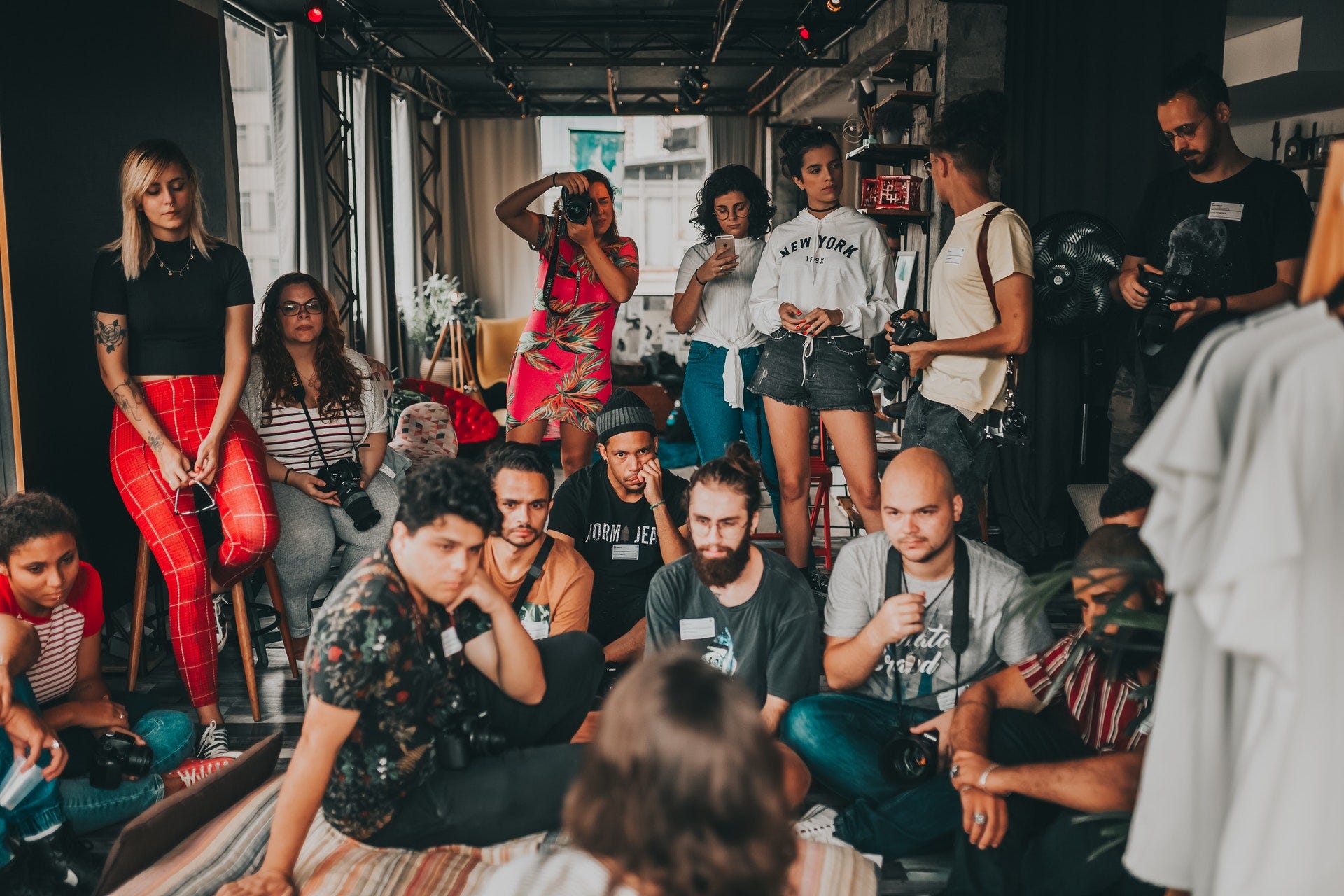
Comments
Post a Comment